The Tech Debt Risk for Early Generative AI Adopters
- Karl Aguilar
- May 3, 2024
- 3 min read
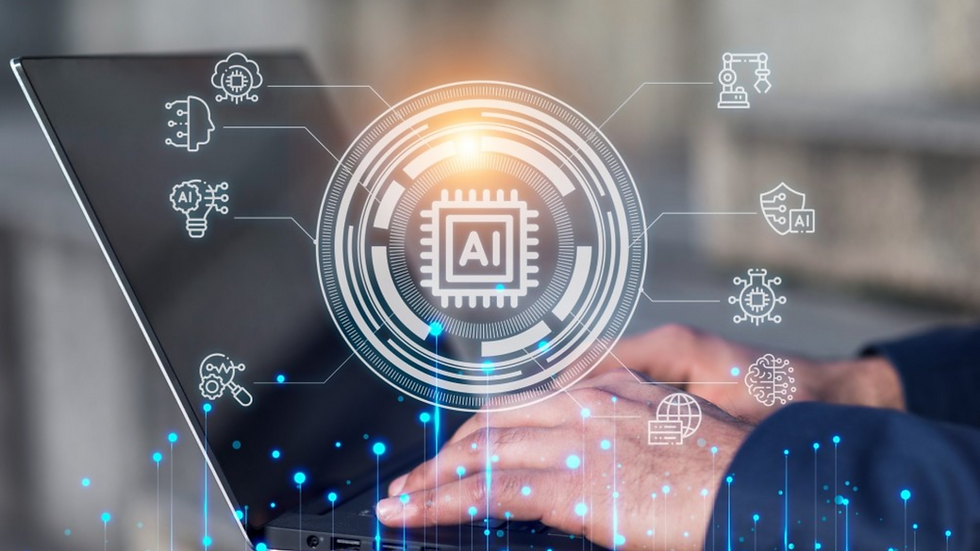
Generative AI has been evolving at a consistently rapid pace alongside its growing usage among enterprises. As such, there has always been the caveat that the generative AI models being used by these enterprises will quickly become outdated.
Given these advancements, any business that has adopted any form of generative AI technology must ensure that the technologies it has adopted is up-to-date in order to remain efficient and competitive not only in its use of generative AI but also in its overall operations. However, maintaining an up-to-date generative AI for the enterprise entails costs that the business may not be prepared for.
Research by Gartner indicates that by 2028, more than half of the enterprises that built custom large language models for their generative AI will abandon initiatives due to costs, complexity, and technical debt. This risk is higher for companies that are “moving fast” in their AI efforts, as well as those who have started at square one.
There is some good news on the horizon though. New techniques and models have emerged recently that have somewhat reduced the cost of generative AI implementation, as well as offering improvements in the technology’s accuracy as well.
But the most effective way for the enterprise looking to escape the technical debt of adopting generative AI is for it to choose an AI mode that is considered “nimble”. What this means for the CIO is that they must choose AI architecture that can be swiftly updated as new updates or versions to the model are released by developers.
Fortunately, many generative AI models in the market have a “nimble” AI architecture. OpenAI, for instance, rolled out GPT-3.5, which pioneered the enterprise generative AI conversation in November 2022 and then launched GPT-4 around two months later, in January 2023. Anthropic rolled out its generative AI chatbot Claude in March 2023 with its first-generation model and made significant updates since. In fact, just this year in March, the third generation model was launched.
While the thought of incurring technical debt in maintaining generative AI models up-to-date might make CIOs recoil, it has been attested that enterprises that are nimble and swift in deploying AI updates have the advantage not only in upskilling initiatives but, more importantly, in acquiring skilled talent to support their AI-driven aspirations. Such technical debt can and should be considered an investment into the enterprise’s future growth.
In fact, it is predicted that by 2027, enterprises will use generative AI tools to create appropriate replacements for legacy apps, which can help lessen modernization costs by 70%. This means that the current costs in up-to-date generative AI yield significant future savings for the enterprise.
Still, some companies are wary of the risks and costs involved of implementing up-to-date generative AI models despite the benefits they offer. As such, CIOs and other company leaders need to balance their organization’s AI goals with risk tolerance. Experts recommend as an interim strategy the use of AI for coding, content generation, or productivity via vendor solutions. Going this route enables a relatively easy procurement and deployment of generative AI throughout the enterprise, offering a lower risk for tech debt as the enterprise prepares its resources for a more long-term commitment towards adopting generative AI in various areas of its operations.
Choosing the right AI model, having a carefully planned AI strategy, and effective gathering and allocation of the necessary resources, can immensely help companies overcome the tech debt risk of generative AI and facilitate a future-ready enterprise.
Comments