Harnessing the Power of the Data Ecosystem
- Karl Aguilar
- Aug 15, 2023
- 3 min read
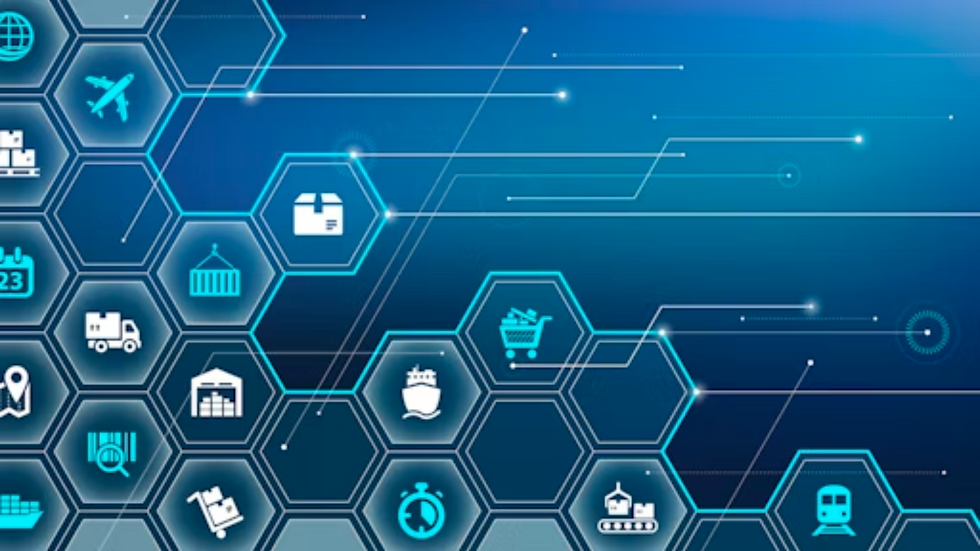
When thinking about a business’s data, your mind may conjure images of spreadsheets, databases, graphs, and charts that are stored either in physical disk space or in a dedicated cloud storage facility. We tend to think of such data on an individual level, which is not surprising as we usually tend to search for and use specific data files for different purposes.
However, treating data on such an individual level misses the forest for the trees, so to speak, ignoring the sheer importance of the plethora of data in possession by an organization and the infrastructure that supports them. and how these data and infrastructures collectively play a vital role in its operations and development.
Because of this, it is important that we think of the collective data that the organization has as a whole, and how each data is connected not only to one another but to the organization itself as part of a greater ecosystem. A data ecosystem if you will.
A data ecosystem refers to a combination of enterprise infrastructure and applications that is utilized to aggregate and analyze information. It enables organizations to better understand their customers and craft superior marketing, pricing, and operations strategies.
If designed correctly, the data ecosystem balances two priorities:
Building economies of scale by attracting participants through lower barriers to entry. In addition, the ecosystem must generate clear customer benefits and dependencies beyond the core product to establish high exit barriers over the long term.
Cultivating a collaboration network that motivates a large number of parties with similar interests (such as app developers) to join forces and pursue similar objectives. One of the key benefits of the ecosystem comes from the participation of multiple categories of players (such as app developers and app users).
The elements of a data ecosystem
A comprehensive and well-designed data ecosystem should consist of the following elements that will ensure its effectiveness. These elements are:
Sensing – This refers to the process of identifying data sources for your project. It involves evaluating the quality of data so you can better understand whether it’s valuable. This evaluation includes asking questions related to its accuracy, validity, completeness, and timeliness.
Collection – This can be completed through manual or automated processes. By default though, as well for greater efficiency and accuracy, data scientists use programming languages to write software designed to automate the data collection process.
Wrangling – This refers to the set of processes designed to transform raw data into a more usable format. Depending on the quality of the data in question, it may involve merging multiple datasets, identifying and filling gaps in data, deleting unnecessary or incorrect data, and “cleaning” and structuring data for future analysis. As with data collection, data wrangling can be performed manually or in an automated fashion. If a dataset is small enough, manual processes will do but for most larger data projects, the amount of data is too vast and requires automation.
Analysis - Depending on the specific challenge your data project seeks to address, this analysis can be diagnostic, descriptive, predictive, or prescriptive. While each of these forms of analysis is unique, they rely on the same processes and tools and require some form of automation, especially when datasets are exceptionally large. After automated processes have been completed, data analysts use their expertise to glean additional insights.
Storage - Data must be stored in a way that’s both secure and accessible in a location or medium dictated by your organization’s data governance procedures.
The benefits of understanding the data ecosystem
Businesses with a good understanding of the data ecosystem and how it works will be able to gain greater insights into the data being stored and how they derive value from it more effectively. This entails different aspects such as process improvements, enhanced customer engagements, and other processes that will ensure higher returns for the enterprise.
At the same time, businesses are able to reduce capital expenses and contain data warehouse costs as data leaders will have better insights into how they can simplify and rationalize the data landscape.
Comentarios